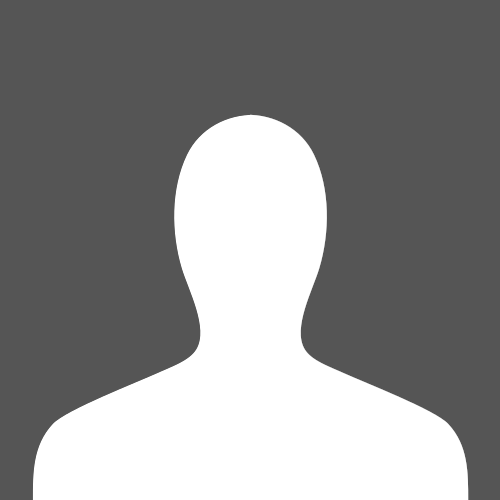
Enzo Ferrante / Demographically-Informed Prediction Discrepancy Index: Early Warnings of Demographic Biases for Unlabeled Populations
Judy Wawira Gichoya / “Shortcuts” Causing Bias in Radiology Artificial Intelligence: Causes, Evaluation, and Mitigation
Abstract
Talk 1: Demographically-Informed Prediction Discrepancy Index: Early Warnings of Demographic Biases for Unlabeled Populations
Abstract: An ever-growing body of work has shown that machine learning systems can be systematically biased against certain sub-populations defined by attributes like race or gender. Data imbalance and under-representation of certain populations in the training datasets have been identified as potential causes behind this phenomenon. However, understanding whether data imbalance with respect to a specific demographic group may result in biases for a given task and model class is not simple. An approach to answering this question is to perform controlled experiments, where several models are trained with different imbalance ratios and then their performance is evaluated on the target population. However, in the absence of ground-truth annotations at deployment for an unseen population, most fairness metrics cannot be computed. In this work, we explore an alternative method to study potential bias issues based on the output discrepancy of pools of models trained on different demographic groups. Models within a pool are otherwise identical in terms of architecture, hyper-parameters, and training scheme. Our hypothesis is that the output consistency between models may serve as a proxy to anticipate biases concerning demographic groups. In other words, if models tailored to different demographic groups produce inconsistent predictions, then biases are more prone to appear in the task under analysis. We formulate the Demographically-Informed Prediction Discrepancy Index (DIPDI) and validate our hypothesis in numerical experiments using both synthetic and real-world datasets. Our work sheds light on the relationship between model output discrepancy and demographic biases and provides a means to anticipate potential bias issues in the absence of ground-truth annotations. Indeed, we show how DIPDI could provide early warnings about potential demographic biases when deploying machine learning models on new and unlabeled populations that exhibit demographic shifts.
Talk 2: “Shortcuts” Causing Bias in Radiology Artificial Intelligence: Causes, Evaluation, and Mitigation
Abstract: Despite the expert-level performance of artificial intelligence (AI) models for various medical imaging tasks, real-world performance failures with disparate outputs for various subgroups limit the usefulness of AI in improving patients’ lives. Many definitions of fairness have been proposed, with discussions of various tensions that arise in the choice of an appropriate metric to use to evaluate bias; for example, should one aim for individual or group fairness? One central observation is that AI models apply “shortcut learning” whereby spurious features (such as chest tubes and portable radiographic markers on intensive care unit chest radiography) on medical images are used for prediction instead of identifying true pathology. Moreover, AI has been shown to have a remarkable ability to detect protected attributes of age, sex, and race, while the same models demonstrate bias against historically underserved subgroups of age, sex, and race in disease diagnosis. Therefore, an AI model may take shortcut predictions from these correlations and subsequently generate an outcome that is biased toward certain subgroups even when protected attributes are not explicitly used as inputs into the model. As a result, these subgroups became nonprivileged subgroups. In this talk, Dr. Gichoya will discuss the various types of bias from shortcut learning that may occur at different phases of AI model development, including data bias, modeling bias, and inference bias. She will also discuss mitigation strategies to bias arising from "shortcuts."
About the Speaker
Enzo Ferrante holds a permanent position as CONICET faculty researcher in Argentina, leading a research line on machine learning methods for biological and medical image analysis at the Applied Artificial Intelligence Laboratory - LIAA (CONICET / Universidad de Buenos Aires) in Argentina. He is also a professor at Universidad Torcuato Di Tella and Universidad de San Andrés, in Buenos Aires, Argentina. His research interests span artificial intelligence and biomedical image analysis, currently focusing on fairness, domain adaptation and generalization, calibration and anatomical segmentation of medical image.
Judy Wawira Gichoya, MD, MS, is an associate professor in the Department of Radiology and Imaging Sciences at Emory University School of Medicine. Dr. Gichoya serves as co-director in leading the Healthcare AI Innovation and Translational Informatics (HITI) Lab. Trained as both an informatician and an interventional radiologist. Her career focus is on validating machine learning models for health in real clinical settings, exploring explainability, fairness, and a specific focus on how algorithms fail. She is heavily invested in training the next generation of data scientists through multiple high school programs, serving as the program director for radiology: AI trainee editorial board and the medical students machine learning elective.